GenFusion
Closing the Loop between Reconstruction and Generation via Videos
- Sibo Wu 1,2
- Congrong Xu 1,3
- Binbin Huang 4
- Andreas Geiger 5
- Anpei Chen 1,5
4University of Hong Kong 5University of Tübingen, Tübingen AI Center
Reconstruction-driven Video Diffusion Model
Artifact-prone Renderings(left) vs Generated RGB-D Videos(right). Our video model efficiently removes reconstruction artifacts and generates realistic content in underobserved regions.
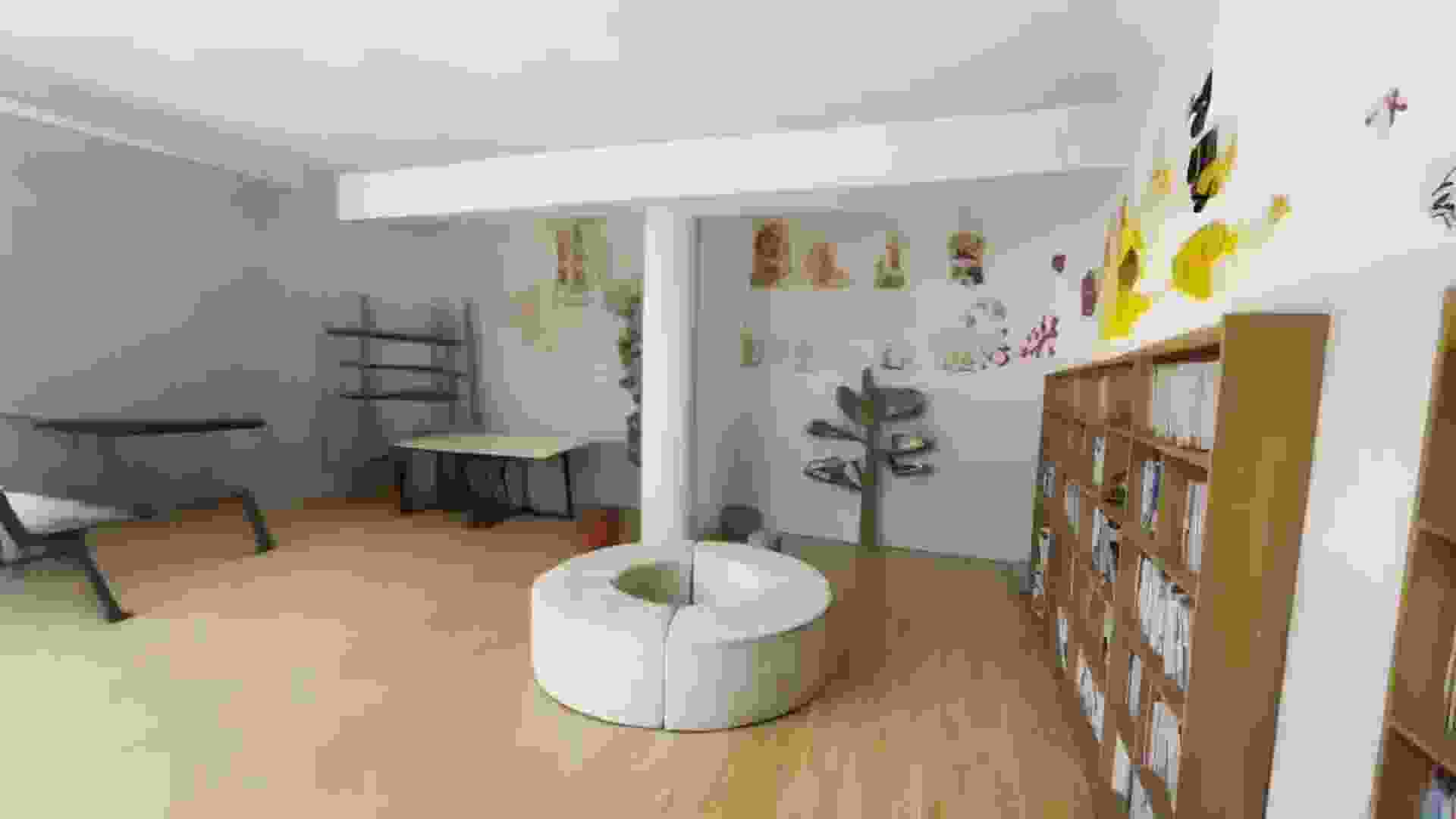
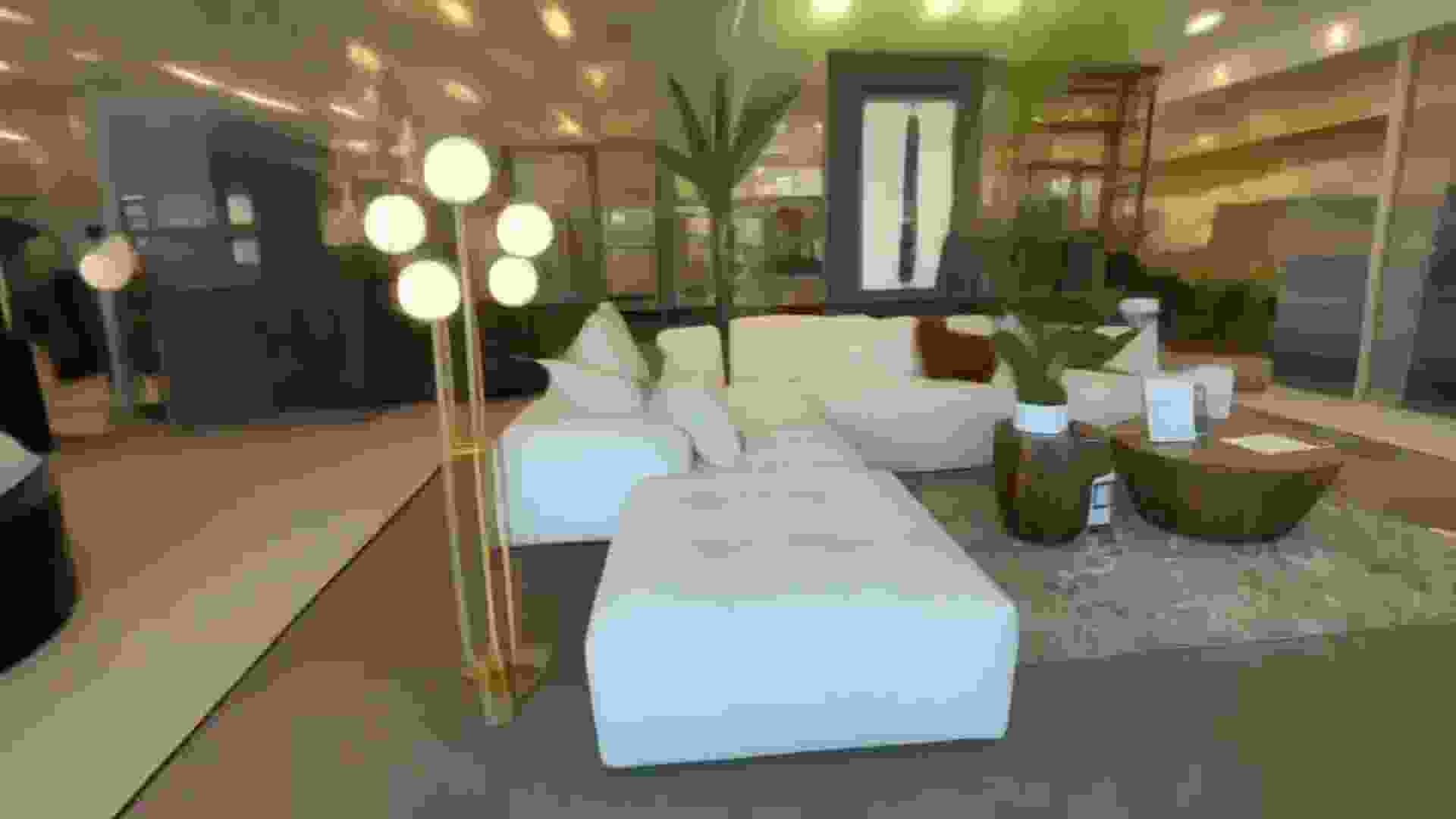
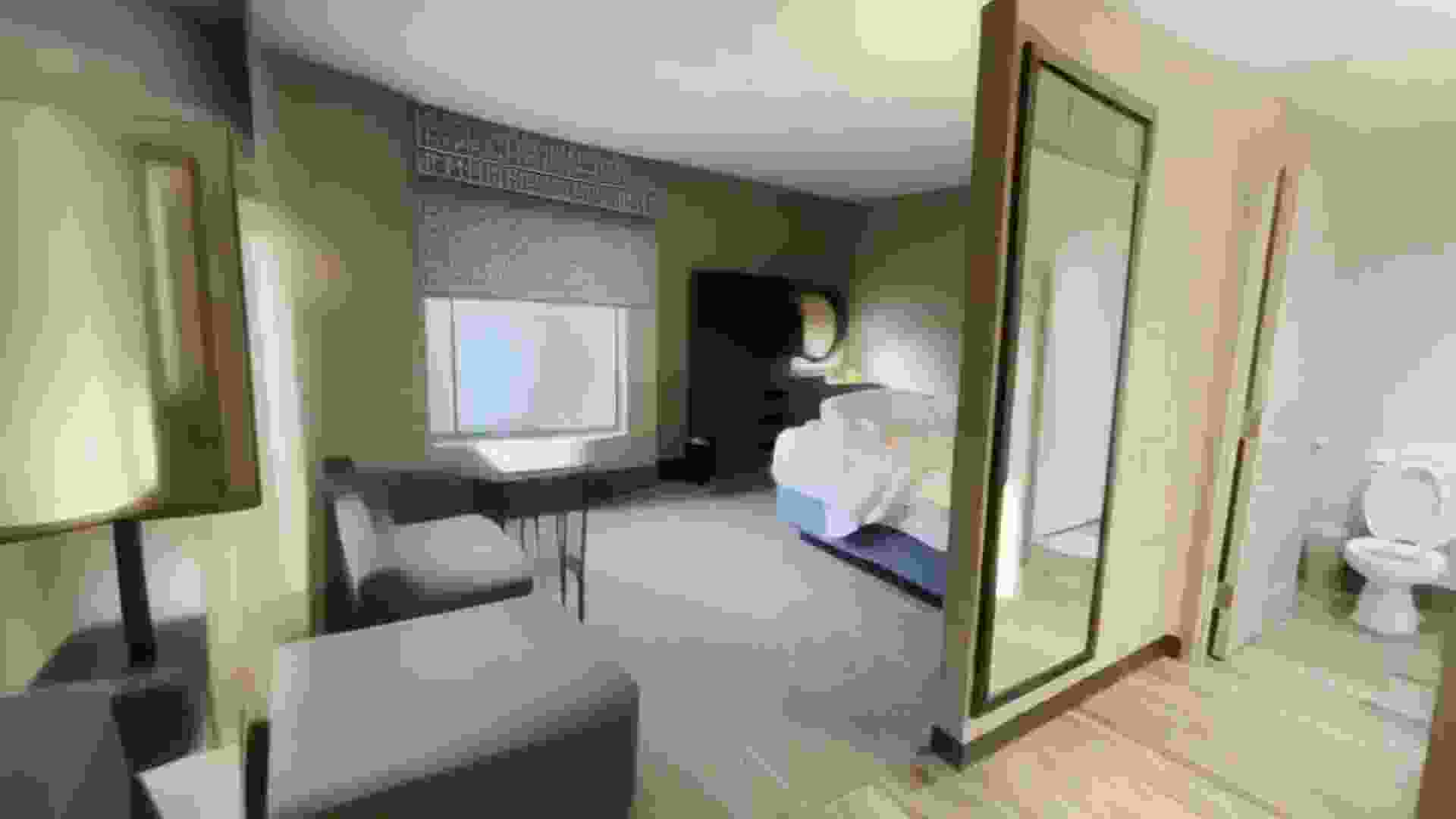
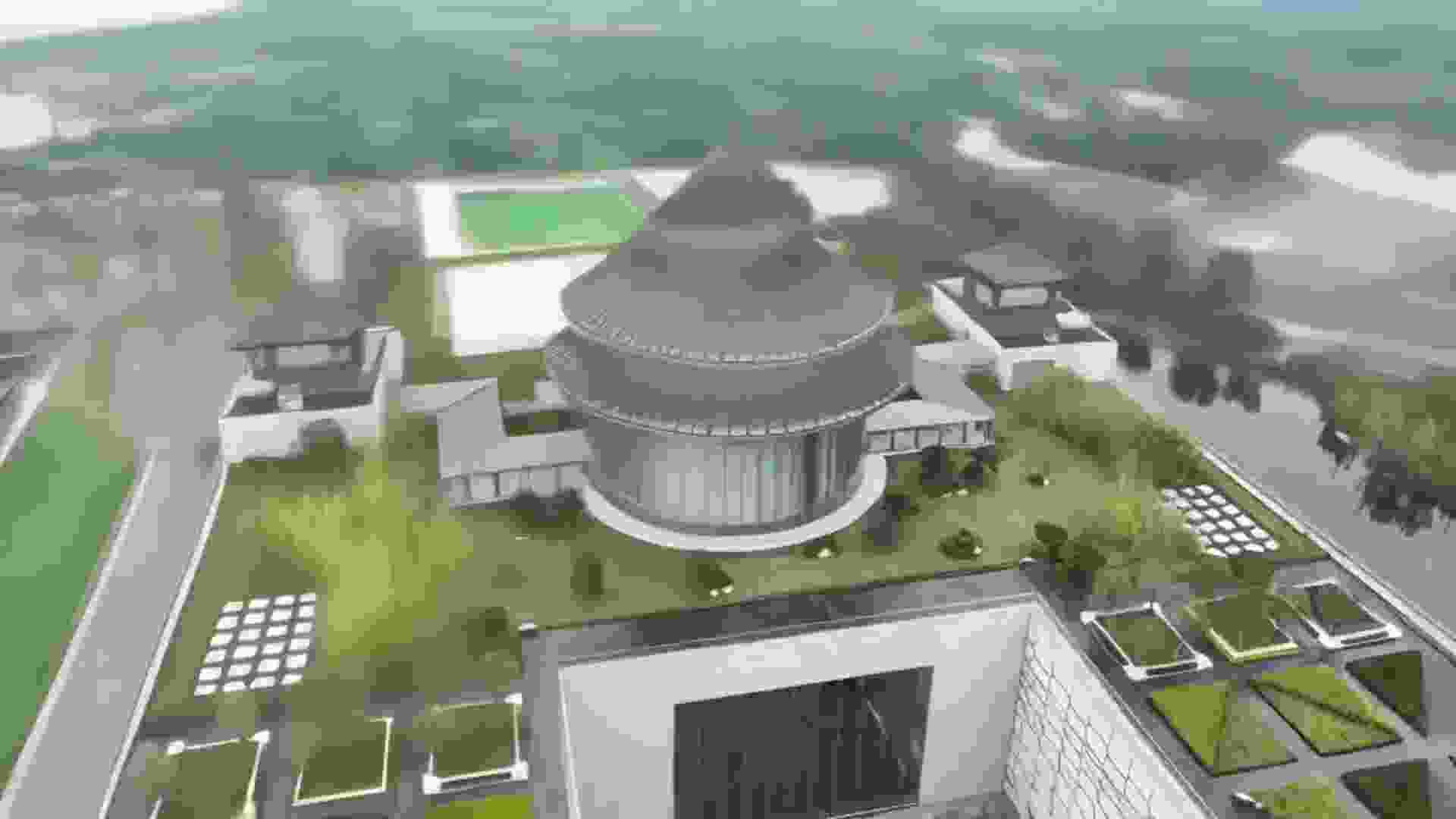
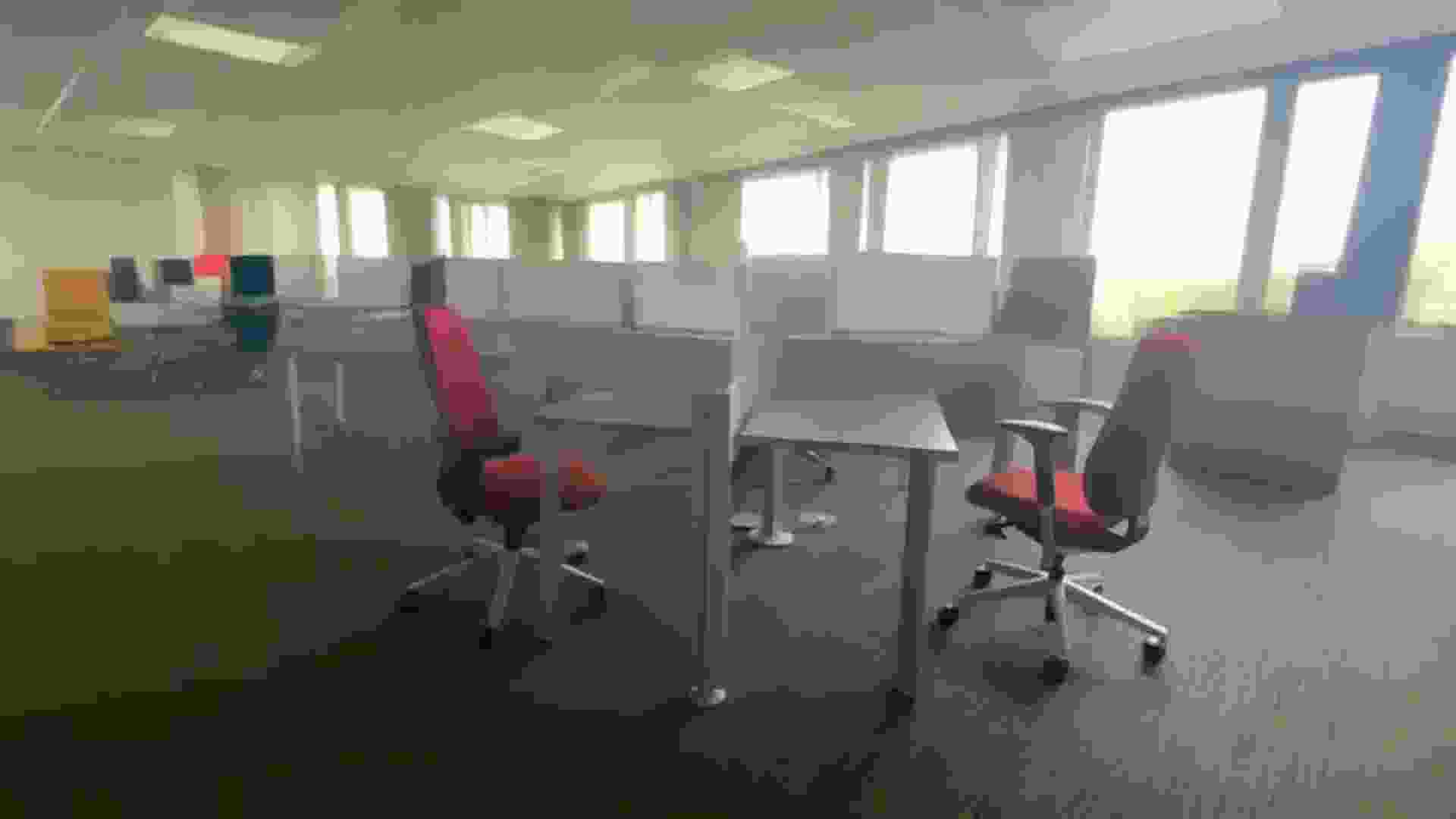
View Extrapolation Results
Baseline (left) vs GenFusion (right). Our approach achieves artifact-free novel view synthesis from narrow field-of-view inputs and outperforms baselines by a significant margin.
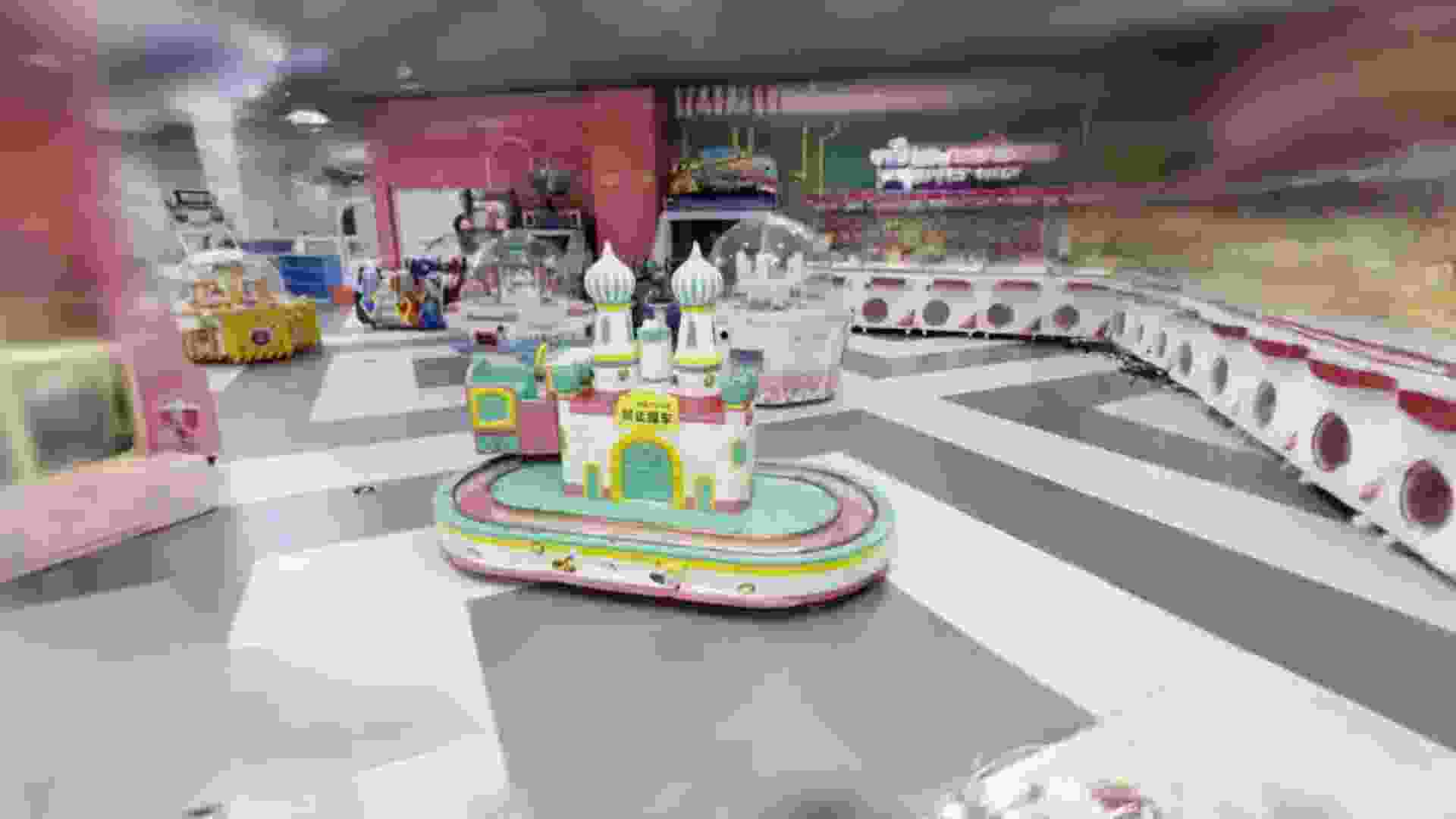
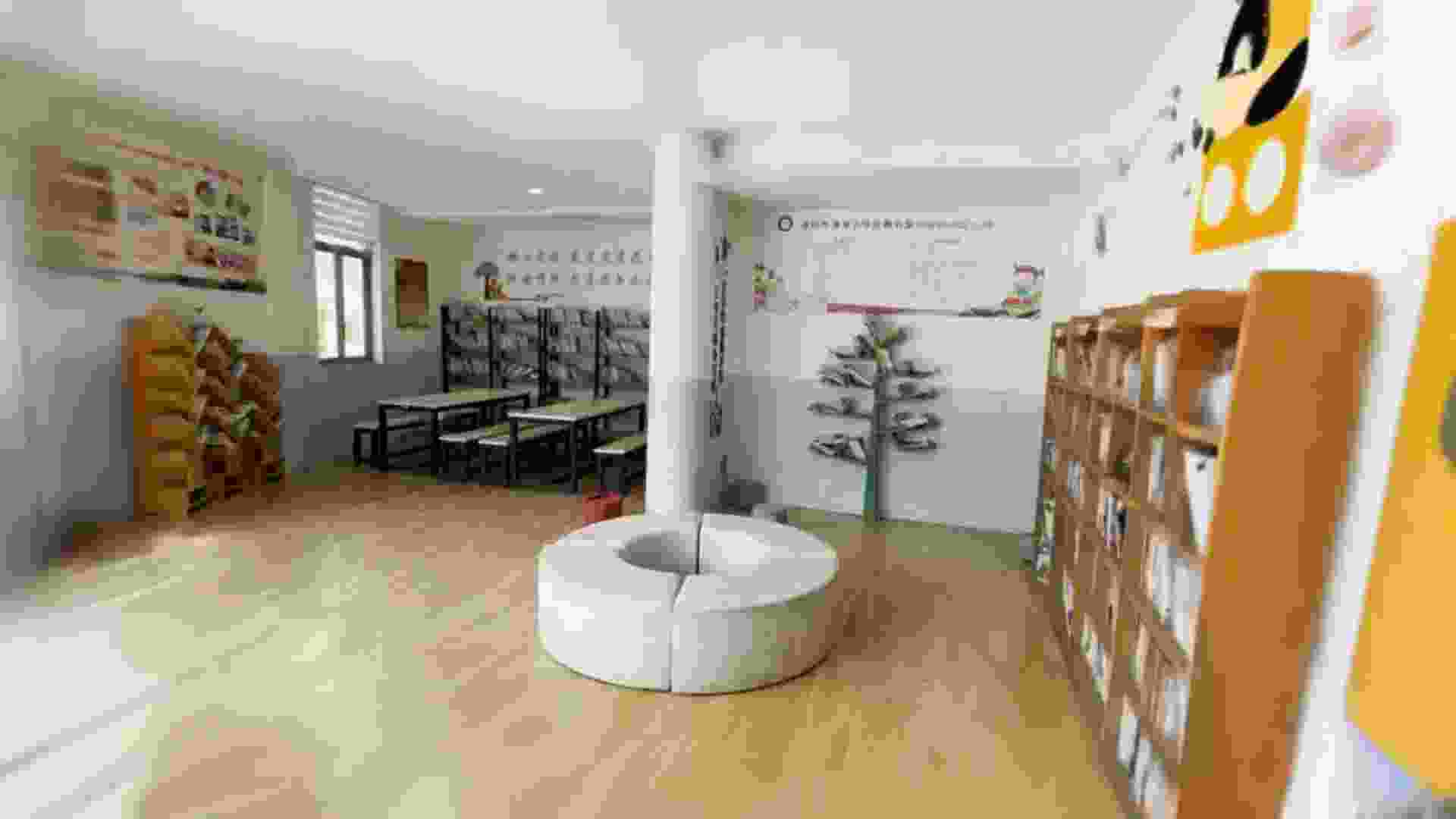
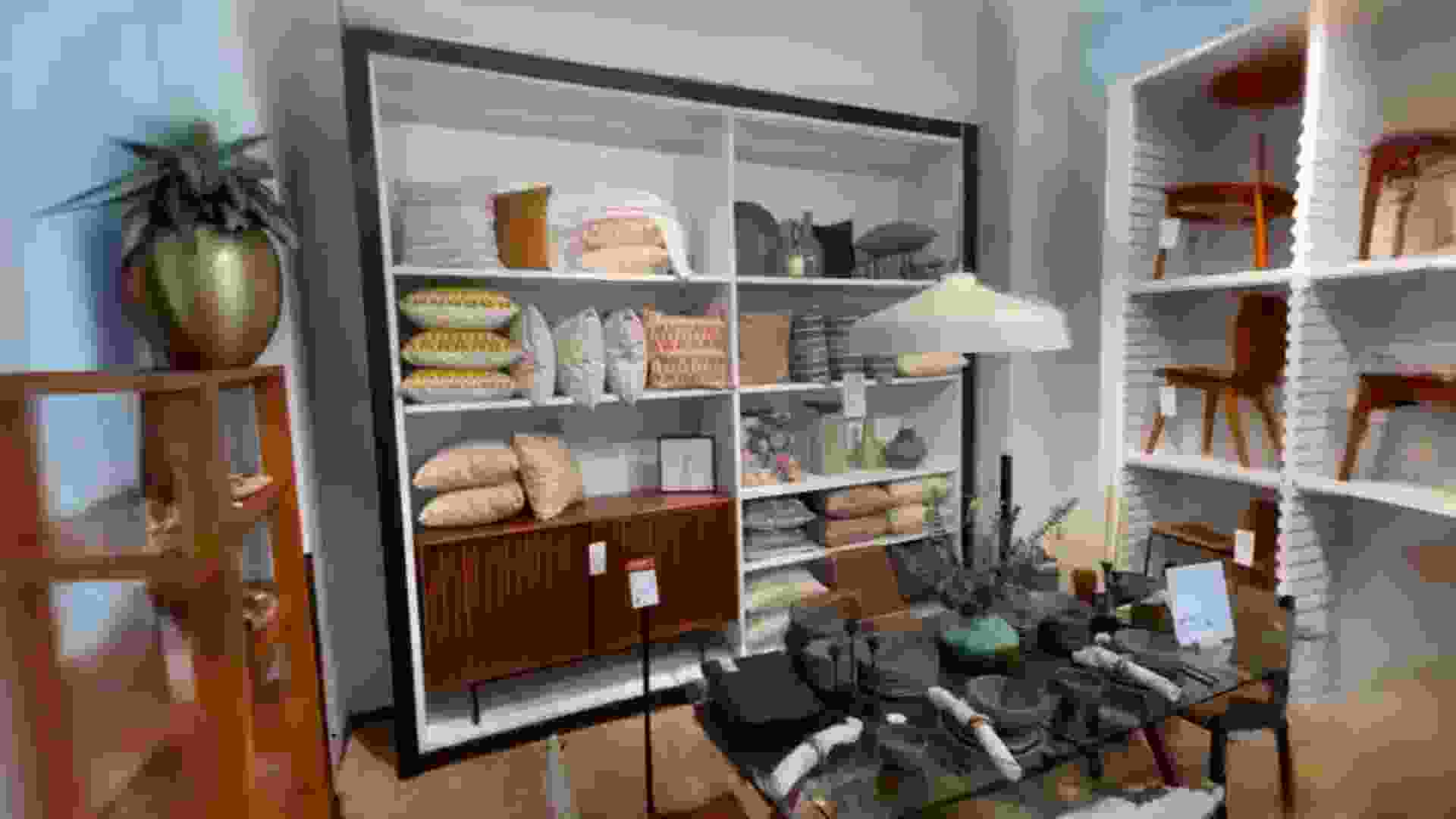
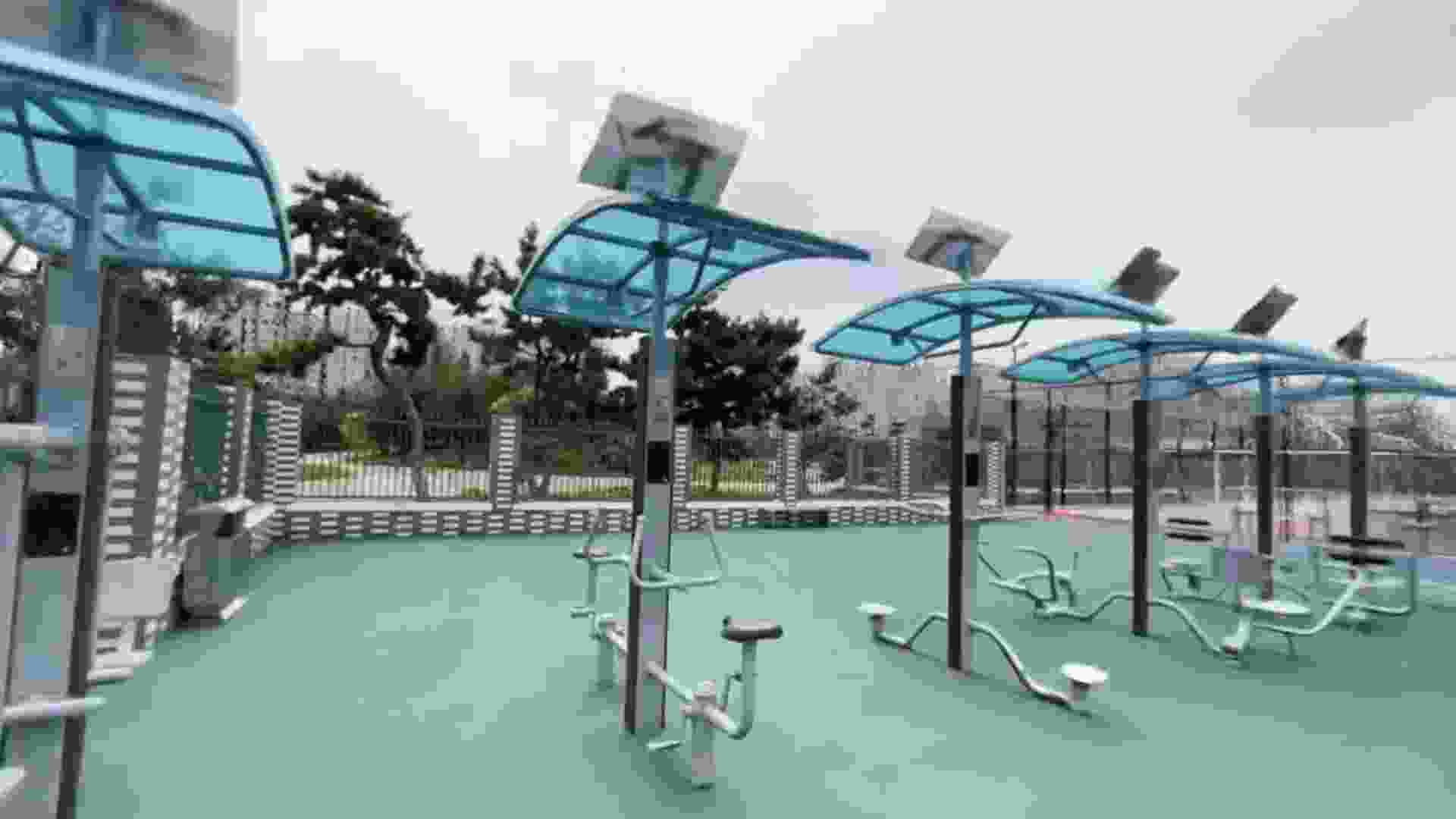
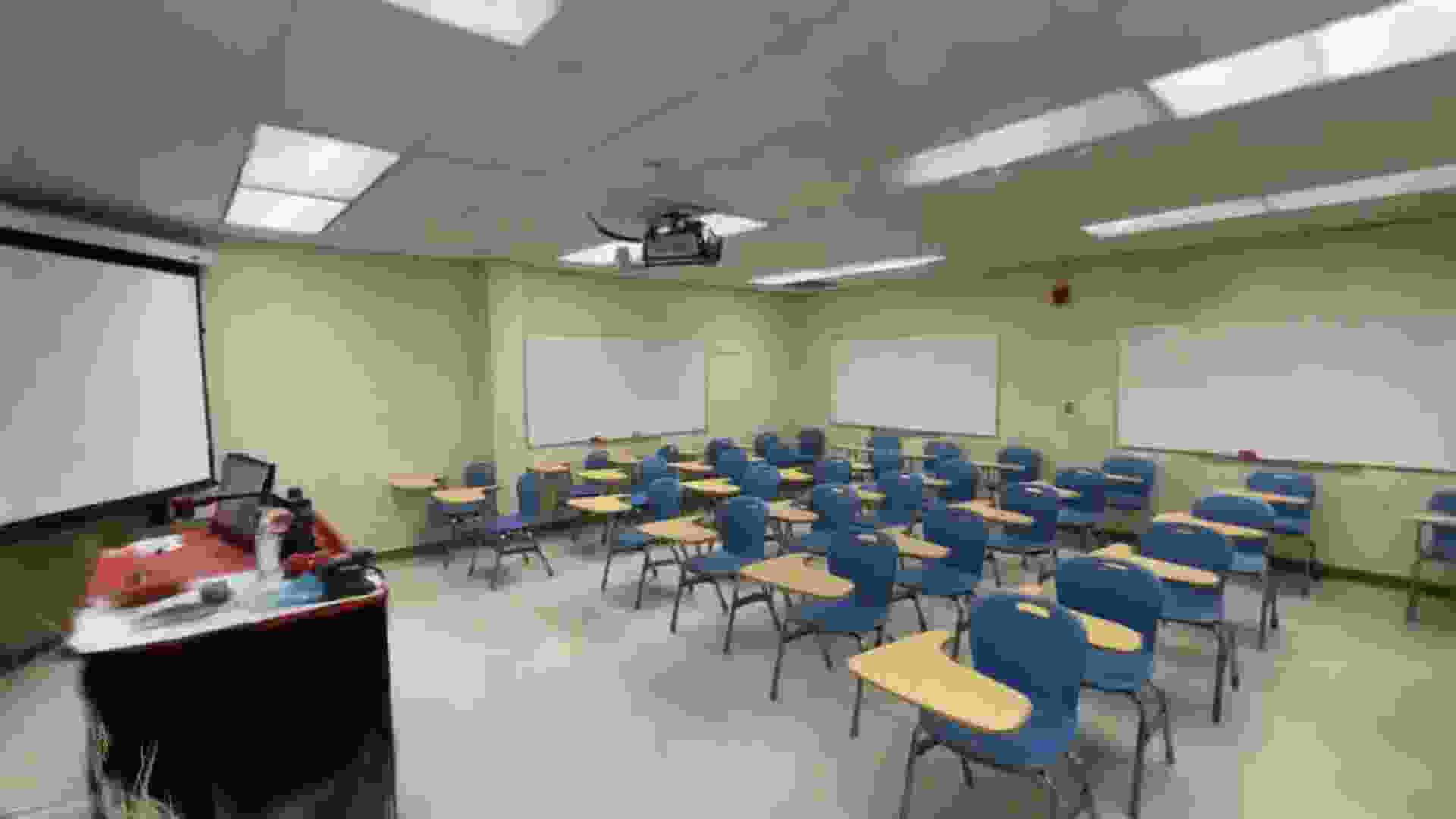
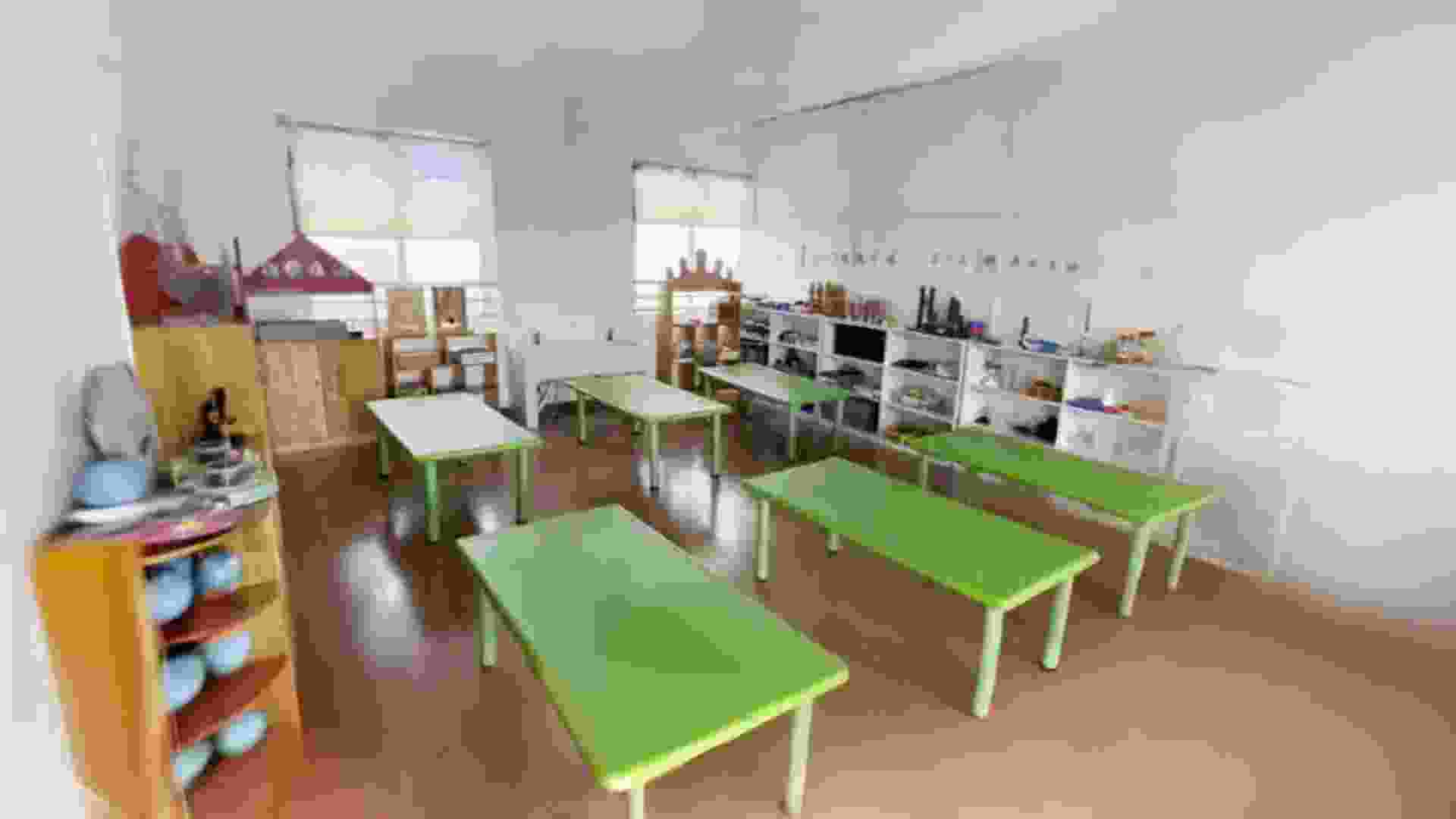
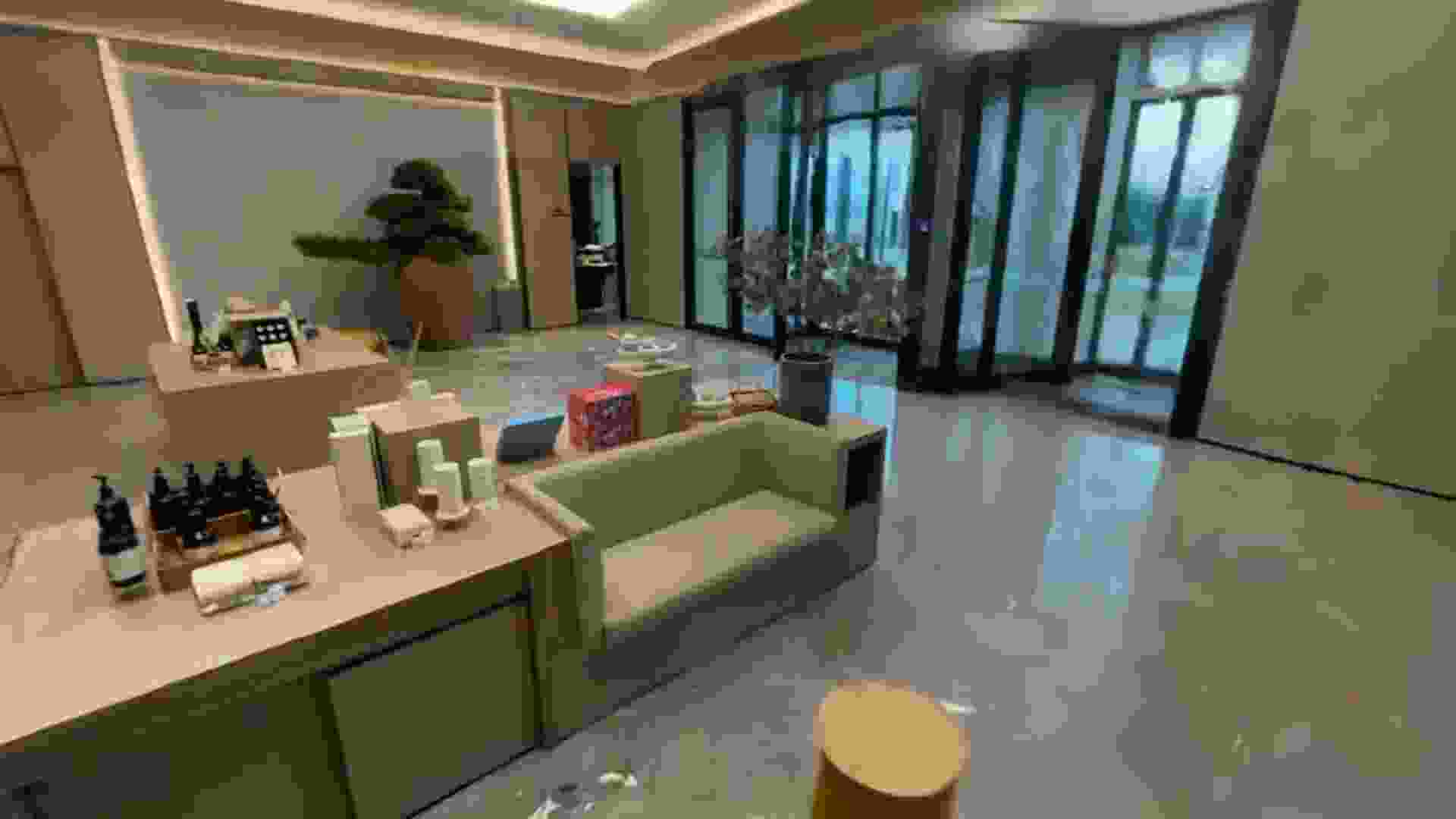
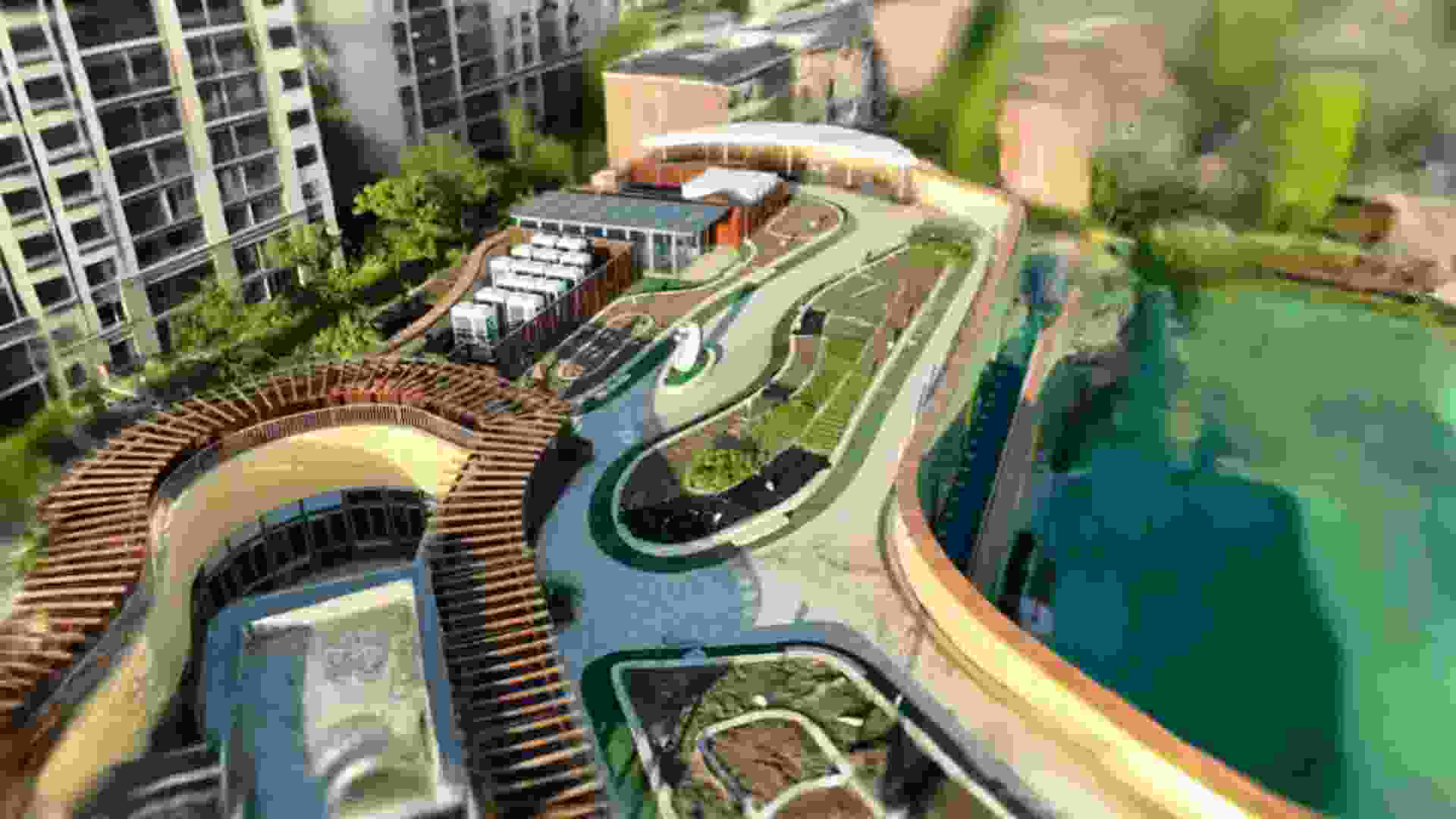
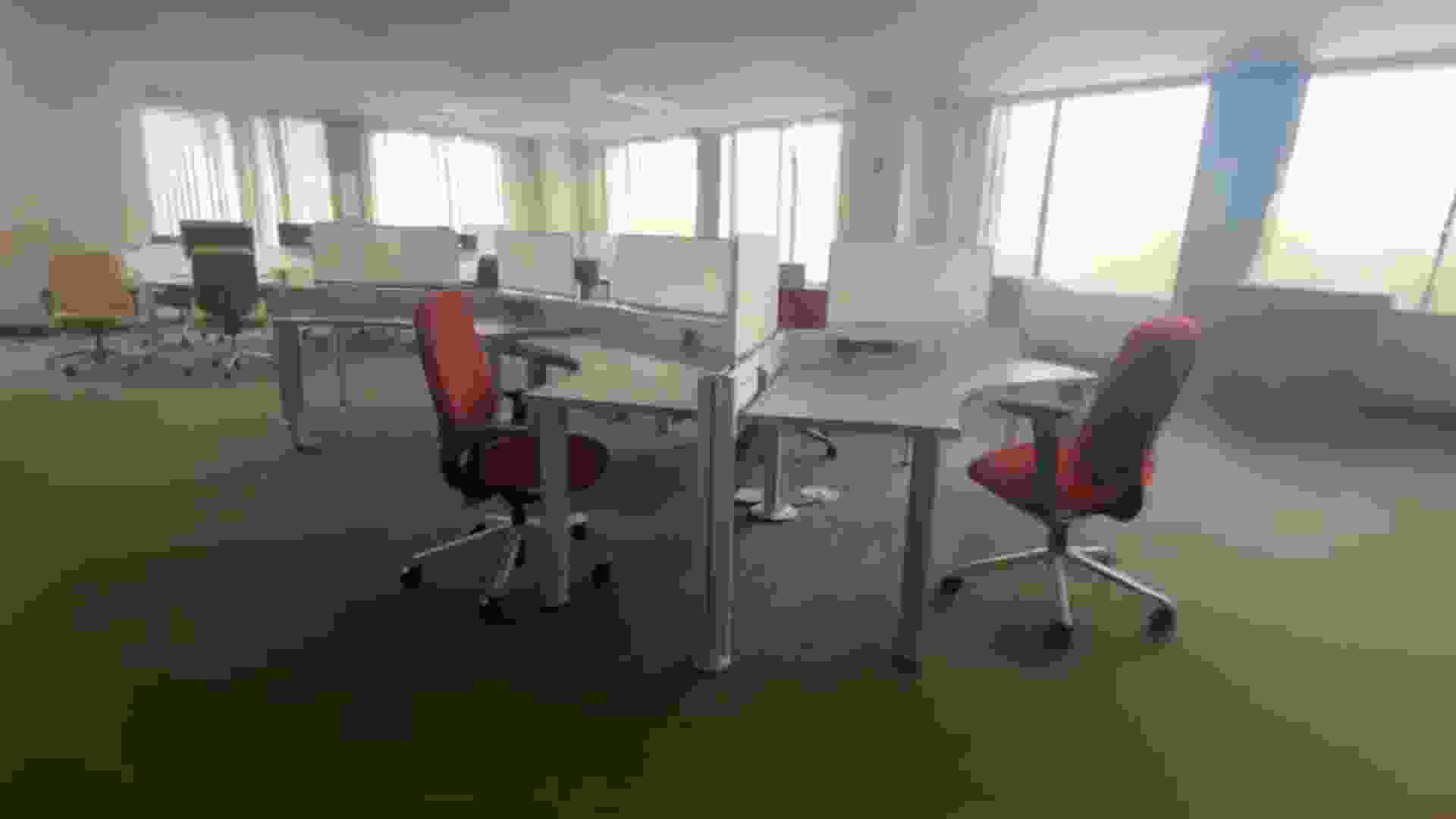
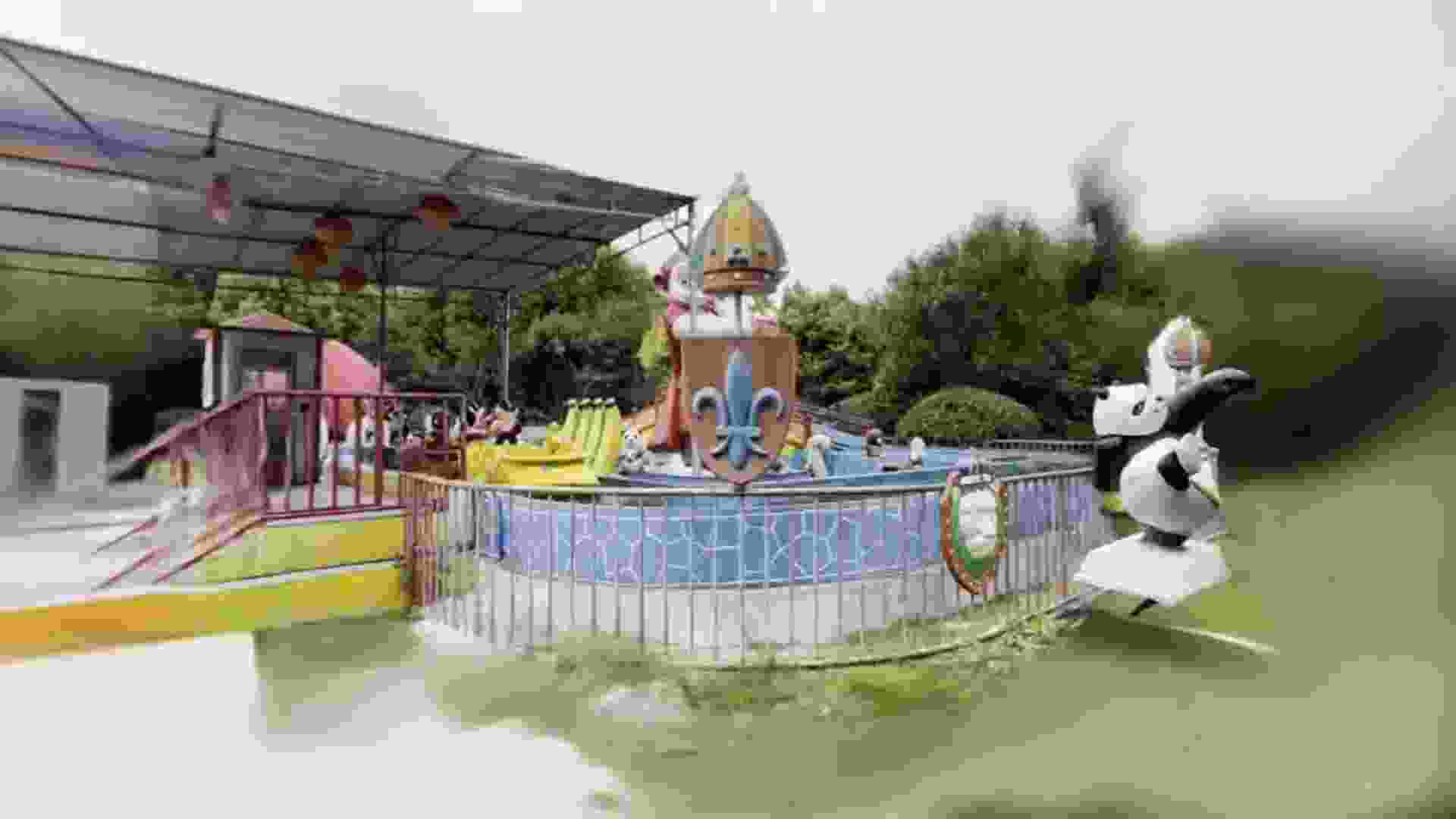
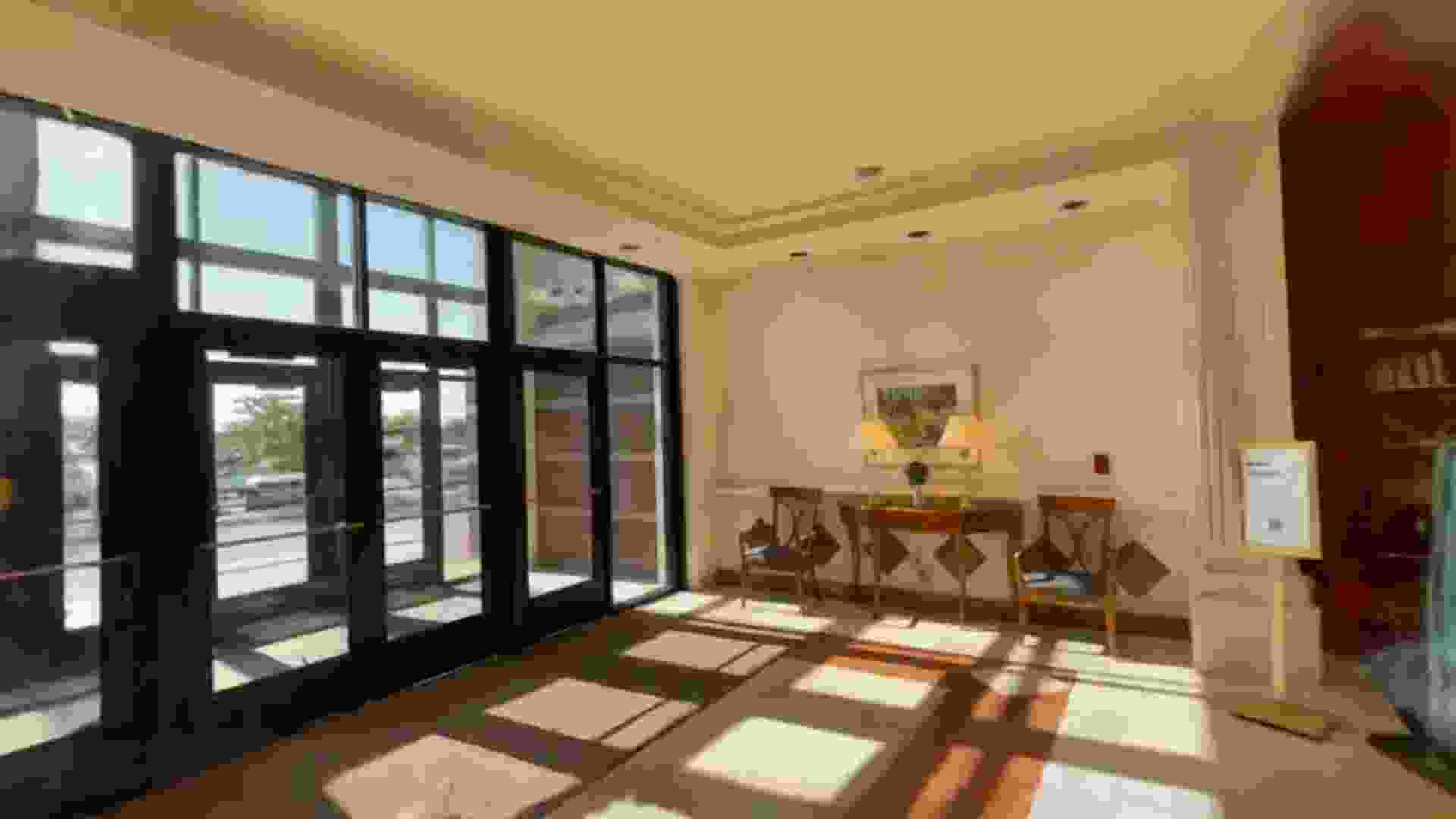
Evaluation of Masked 3D Reconstruction Metrics
Abstract
Recently, 3D reconstruction and generation have demonstrated impressive novel view synthesis results, achieving high fidelity and efficiency. However, a notable conditioning gap can be observed between these two fields, e.g., scalable 3D scene reconstruction often requires densely captured views, whereas 3D generation typically relies on a single or no input view, which significantly limits their applications. We found that the source of this phenomenon lies in the misalignment between 3D constraints and generative priors. To address this problem, we propose a reconstruction-driven video diffusion model that learns to condition video frames on artifact-prone RGB-D renderings. Moreover, we propose a cyclical fusion pipeline that iteratively adds restoration frames from the generative model to the training set, enabling progressive expansion and addressing the viewpoint saturation limitations seen in previous reconstruction and generation pipelines. Our evaluation, including view synthesis from sparse view and masked input, validates the effectiveness of our approach.
Gen Fusion = Video Generation + RGB-D Fusion
Our approach contains two stages: video diffusion pre-training (left) and zero-shot generalization (right). In pre-training, we first fine-tune a RGB video diffusion model on RGB-D videos from a large-scale real-world DL3DV-10K video dataset. Captured videos are patchified, and a random patch sequence is selected for 3D scene reconstruction, rendering full-frame RGB-D videos as input to our video diffusion model, supervised by the original video capture and its monocular depth. During generalization, we treat reconstruction and generation as a cyclical process, iteratively adding restoration frames from the generative model to the training set for artifact removal and scene completion.
Experiments
Our experiments evaluate both view interpolation and extrapolation scenarios, using sparse view reconstruction for interpolation and masked reconstruction for extrapolation. Furthermore, we conduct experiments on scene completion to demonstrate the framework's generalization ability.
View Interpolation Results
Mip-NeRF360 scene trained on 3 views
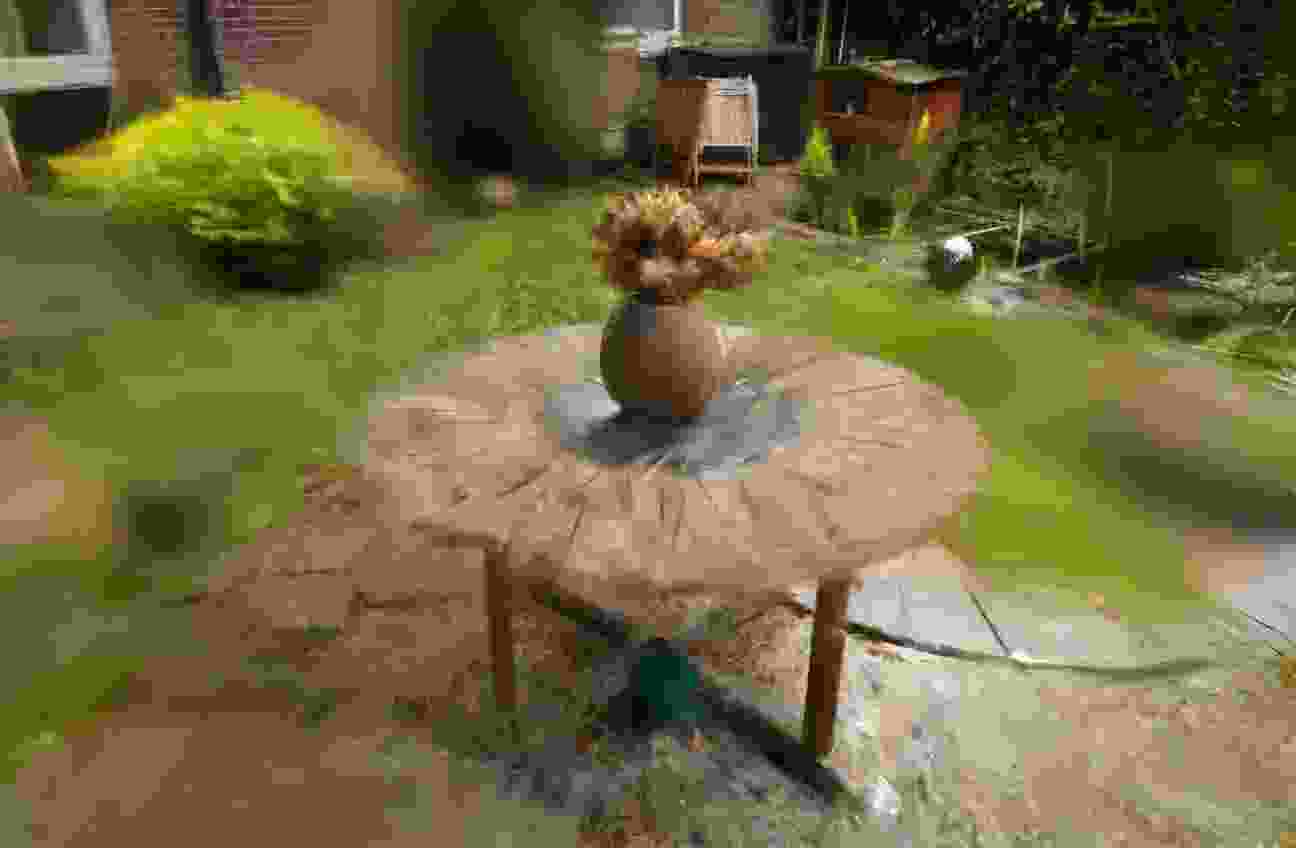
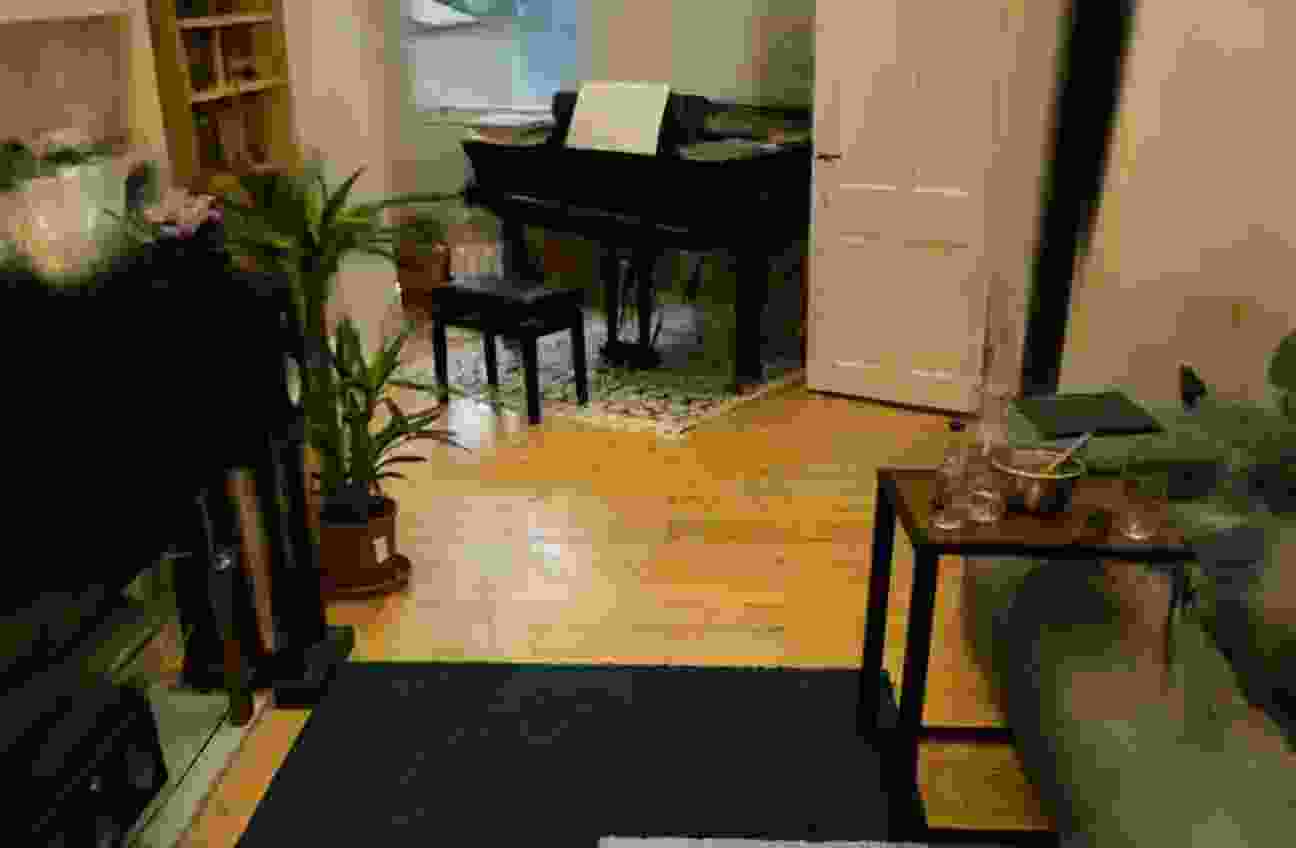
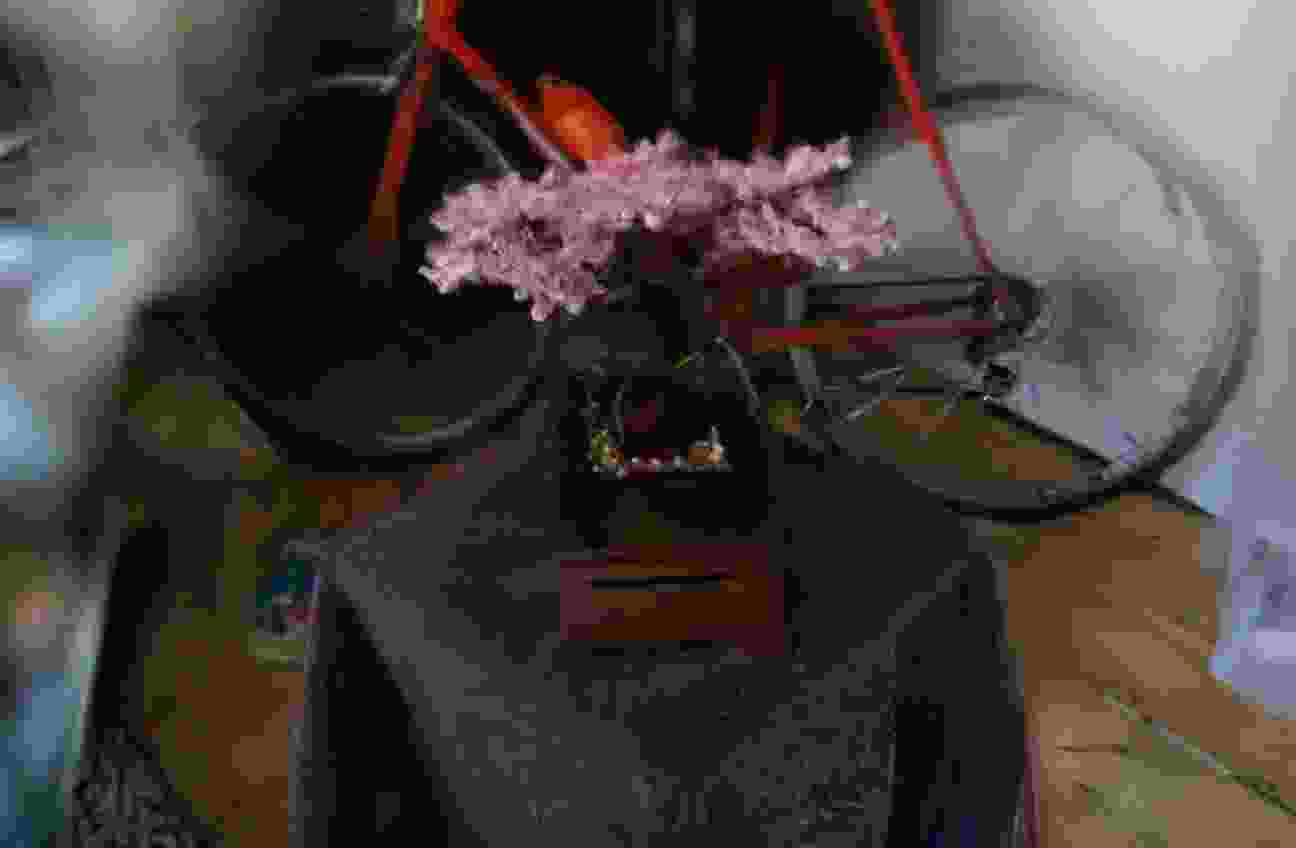
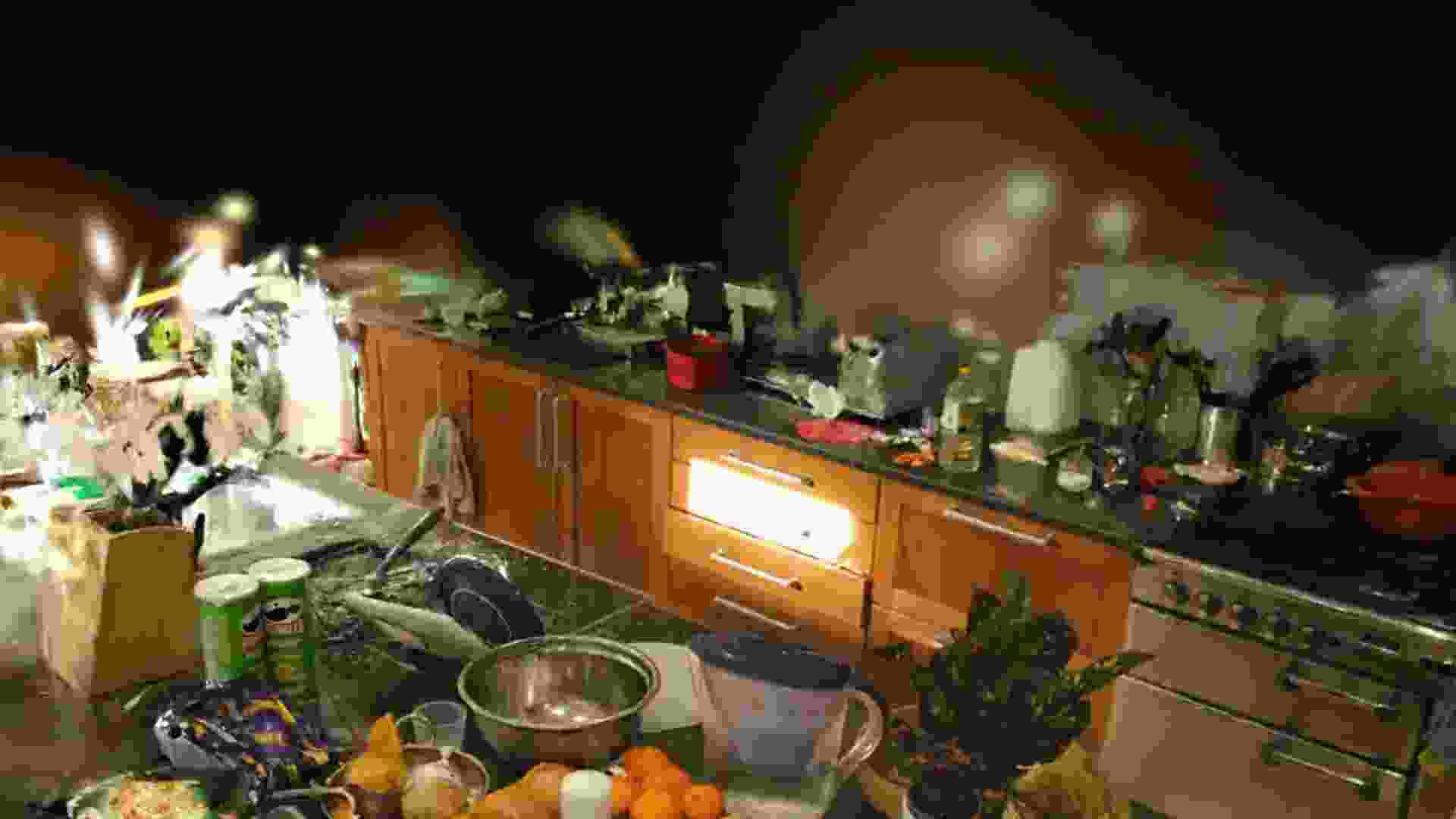
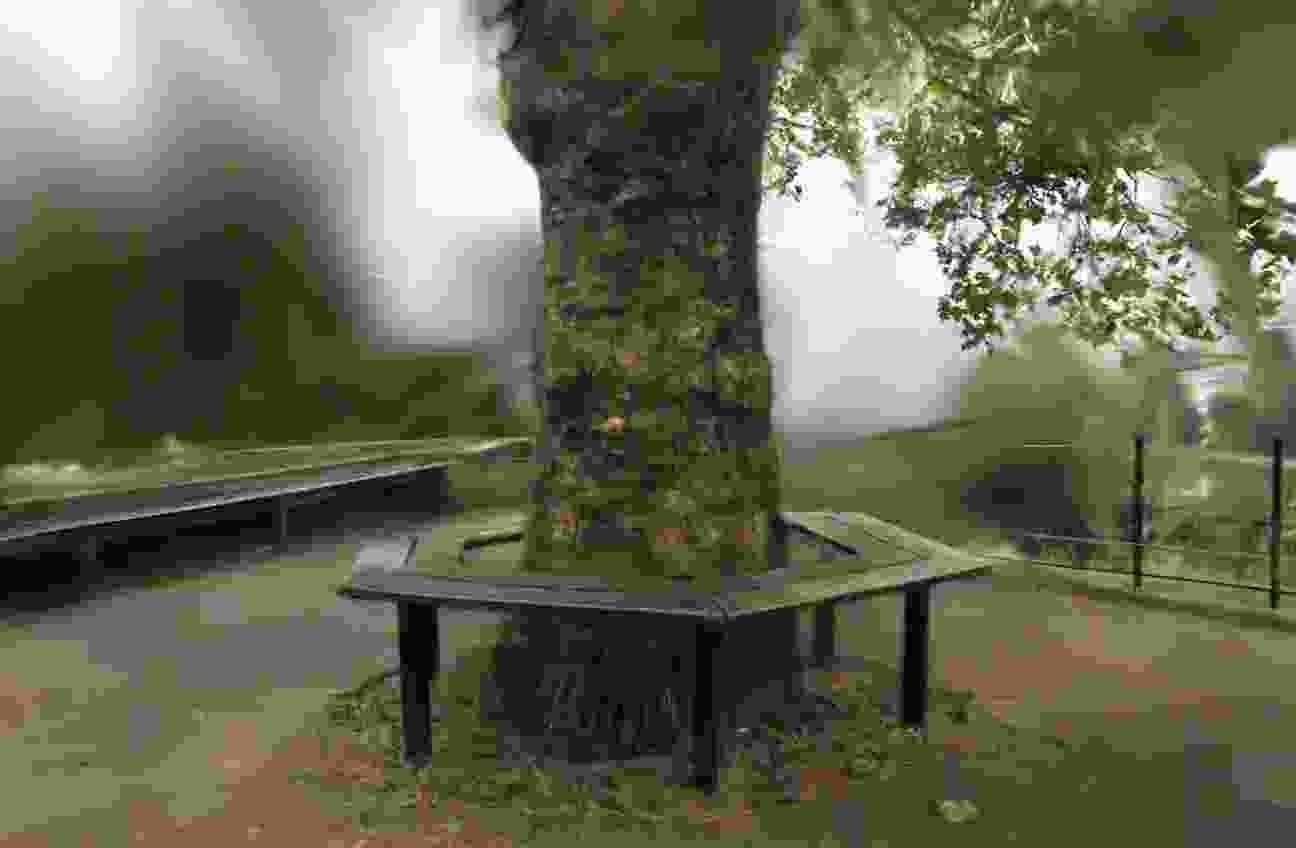
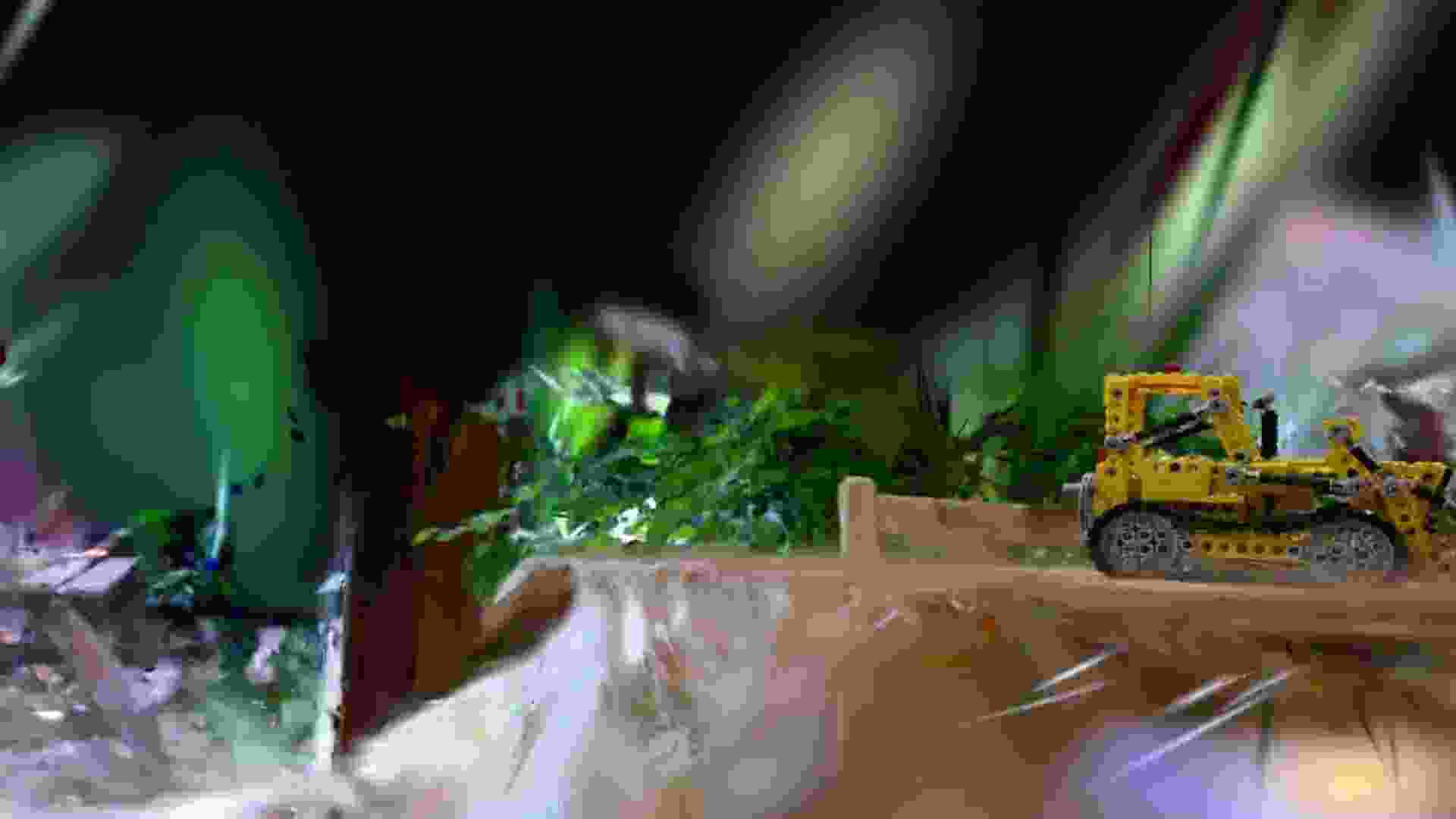
Evaluation of Sparse View 3D Reconstruction Metrics (Mip-NeRF360)
Scene Completion Results
Scene-level completion on unobserved region
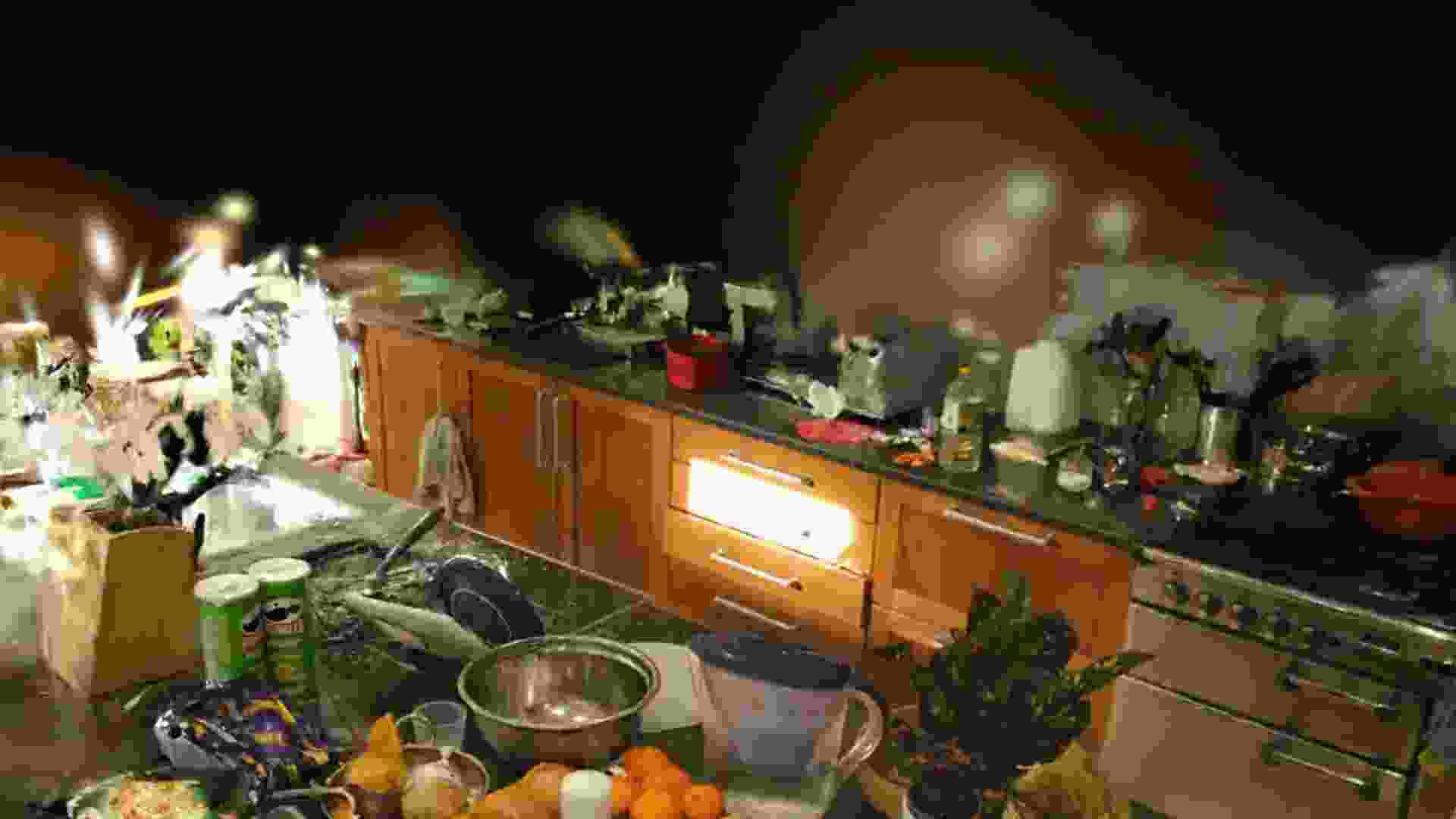
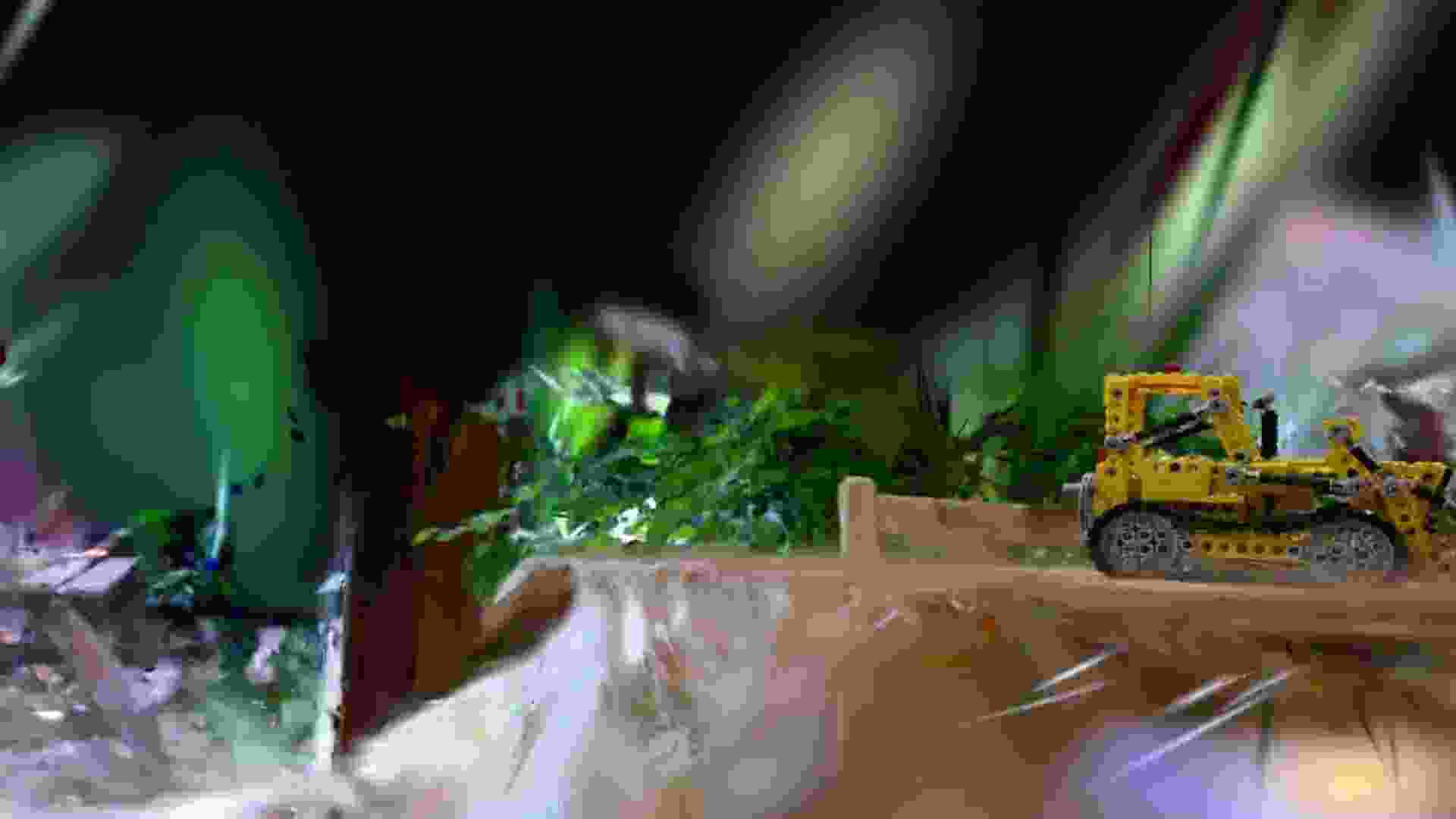
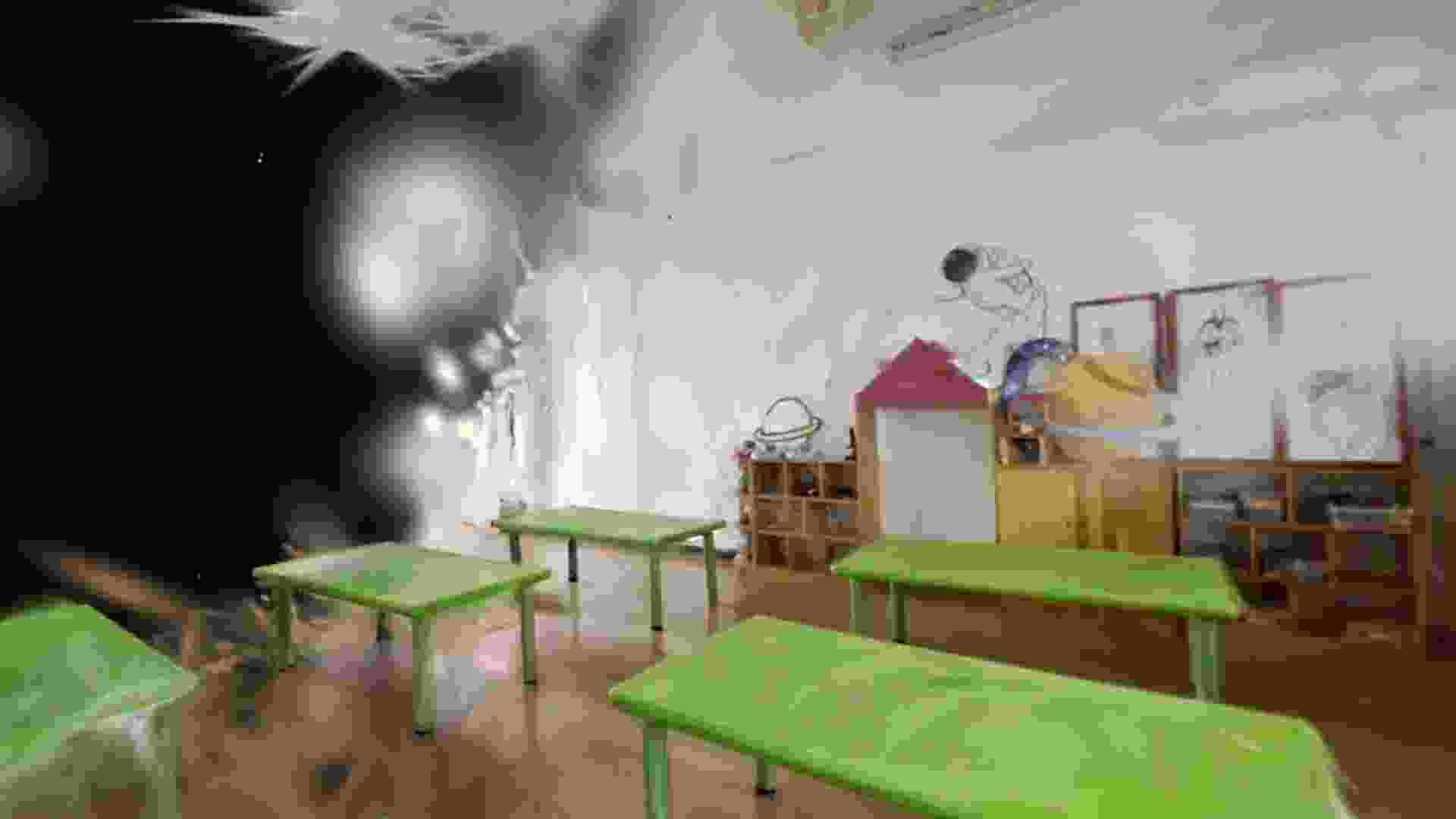
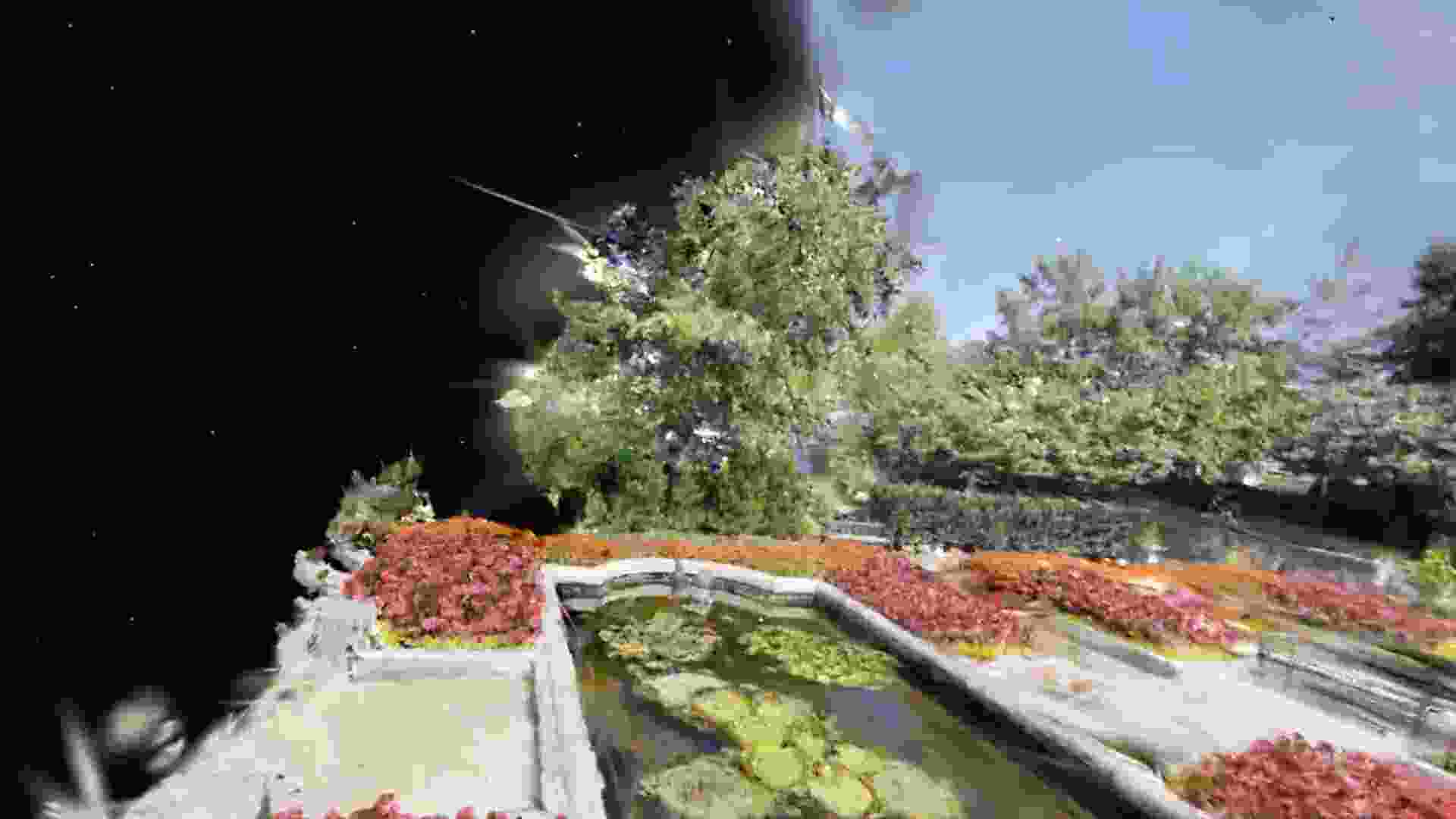
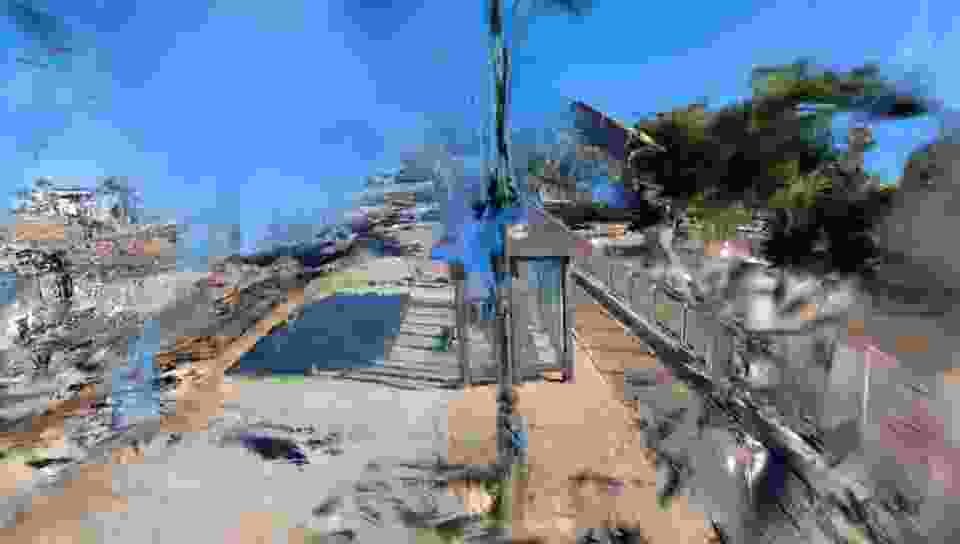
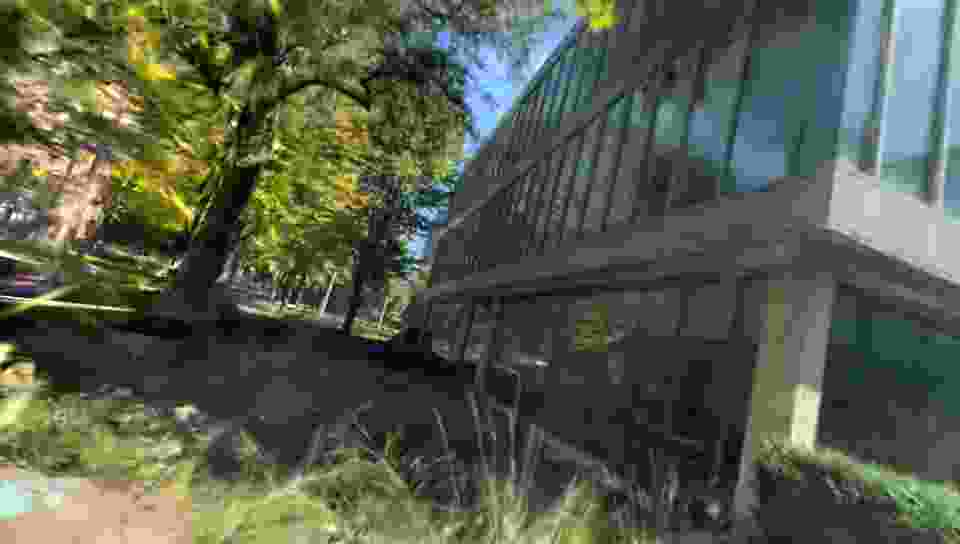
Acknowledgements
-
This website is based on the Video
Compare project from Liangrun Da.